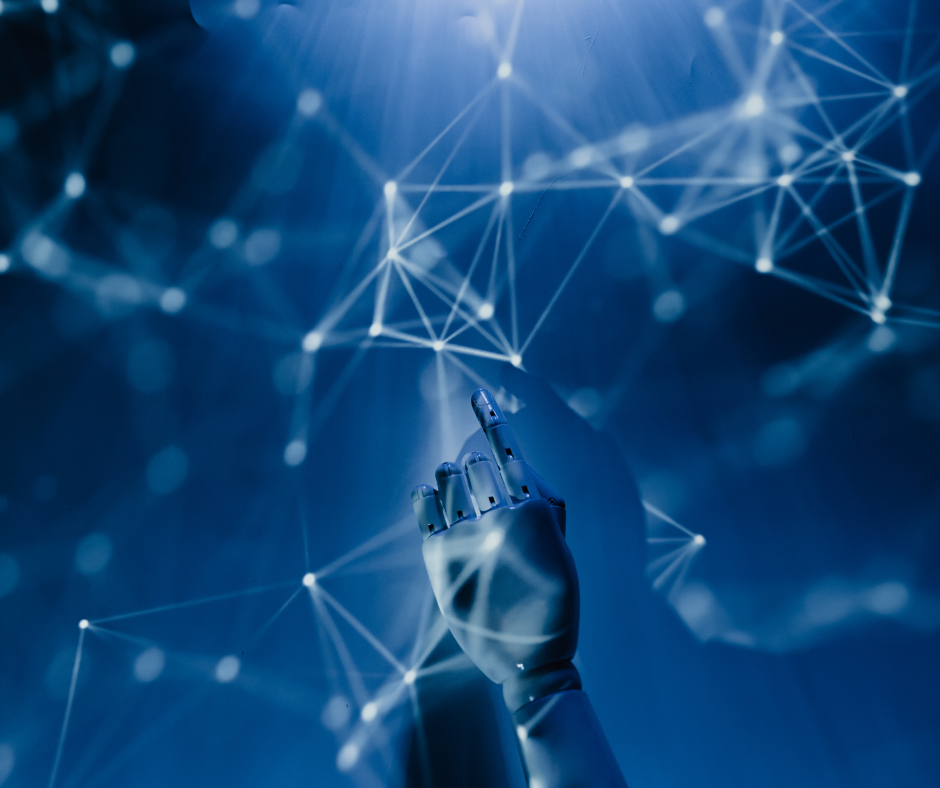
How AI Integrates with Snowflake
AI integration with Snowflake involves leveraging Snowflake’s data warehousing capabilities to support AI-driven analytics and machine learning workflows. Below are the relevant components to the integration including data accessibility, data preparation and transformation, data integration and consolidation, scalability and performance, collaboration and data sharing, integration with AI tools and real-time analytics. Each are important to your business in impactful ways.
Operational Efficiency
Quality data is essential for efficient business operations. Inaccurate or incomplete data can lead to errors, delays, and inefficiencies in processes such as order management, inventory control, and supply chain management. Accurate data ensures smooth operations and supports process optimization.
Data Accessibility
Snowflake provides a centralized and scalable data repository, making it easier to access and analyze large volumes of data. This accessibility is crucial for AI initiatives as AI models typically require vast amounts of data for training and analysis.
Data Preparation and Transformation
Snowflake’s data engineering capabilities allow users to preprocess and transform data. AI models often require clean, structured, and appropriately formatted data. Snowflake’s ability to handle data transformation tasks supports data preparation for AI workflows.
Data Integration and Consolidation
Snowflake supports data integration from various sources, including data lakes, databases, and third-party systems. This capability enables organizations to consolidate their data into a unified view, making it easier to feed data into AI models and perform comprehensive analyses.
Scalability and Performance
AI applications, especially those involving large-scale machine learning, require significant computational power and storage capabilities. Snowflake’s cloud-based architecture allows users to scale up or down compute resources as needed, ensuring optimal performance for AI workloads.
Collaboration and Data Sharing
Snowflake’s collaborative environment facilitates sharing and collaboration on data and AI models across teams. This is crucial for data scientists, engineers, and analysts working on AI projects, allowing them to access and share data, code, and insights seamlessly.
Integration with AI Tools
Snowflake integrates with popular AI tools and frameworks, such as Python libraries like TensorFlow, PyTorch, and scikit-learn. This integration enables data scientists and developers to leverage their preferred AI tools while harnessing the power of Snowflake’s data warehousing capabilities.
Real-time Analytics
Snowflake’s ability to handle real-time data ingestion and processing is valuable for AI applications that require timely insights and predictions. Real-time data integration and analysis enable organizations to deploy AI models that respond to dynamic and rapidly changing data streams.
The integration of AI with Snowflake empowers organizations to unlock the full potential of their data by leveraging advanced analytics and machine learning capabilities. It facilitates data-driven decision-making, predictive modeling, anomaly detection, personalization, and other AI-driven applications that drive business value and innovation.
Ready to discuss how to simplify your journey to becoming a data driven business?